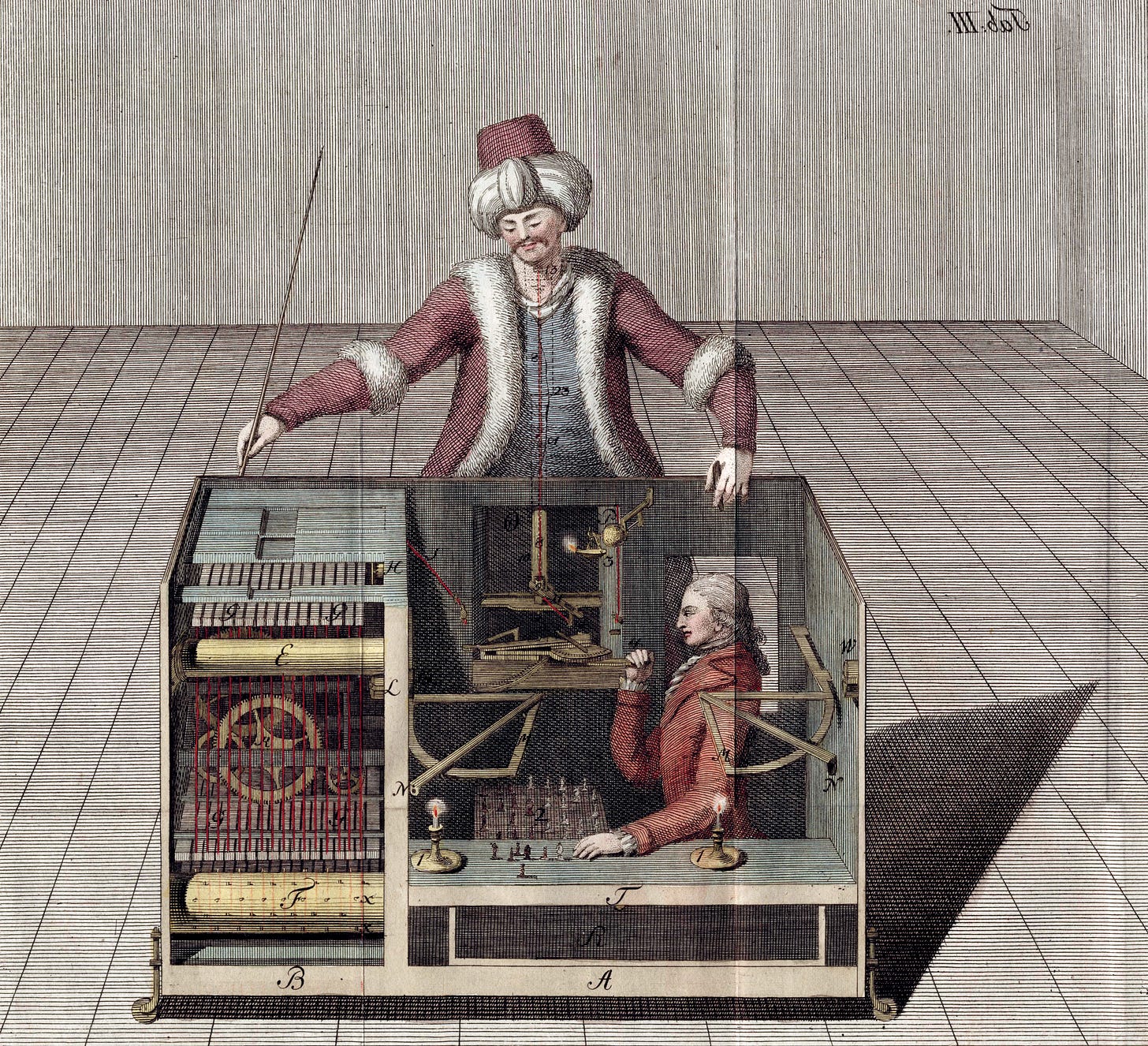
For nearly a century, the Mechanical Turk was seen as an automated marvel—a machine that somehow mastered the game of chess to the point it could beat grandmasters throughout Europe and America. It must have been amazing and terrifying to watch a machine match wits with and often outplay a human being in a game of strategy. Could you imagine watching it by lamplight alone? There were no electric lights when it first appeared in 1770. Thomas Edison wasn’t even born yet!
But the Turk was just an illusion. A man hid inside the machine. By the time the Mechanical Turk was revealed as a hoax, many of the people who’d watched it had already died. For 84 years the device and its illusion captivated audiences. Sure, there were many who suspected that what they were watching was an illusion, including Edgar Allan Poe, but many others believed it was true.
Now generative AI can reason, or so it seems. We’ve been given a glimpse at advanced large language model processes through reasoning traces found in models by OpenAI and DeepSeek. When we use one of these new reasoning models, we are often invited to view its process—its thinking. But just like the automatons of centuries ago, we’re being invited to suspend our disbelief and buy a fantasy of sorts. No, so-called reasoning models don’t think, nor do they reason as we do, but the illusion that they can is key to creating a narrative that there’s more than just a machine we’re using.
Behind the Curtain of AI Reasoning
Derek Lowe’s An Evaluation of “Deep Research” Performance analyzes OpenAI’s newest toy and finds competent results mixed with subtle errors, omissions, and outright faults that look and sound quite convincing but don’t hold up under scrutiny. For one, Lowe notes the model doesn’t favor recent scholarship around his topic and views them with the same weight as sources that are decades old. Another issue is the model confused terms throughout the output, muddling the result. All of these simple errors are noted before Lowe talks about the big one—Deep Research ended up missing the connection a human would have made. Lowe sums up his evaluation:
As with all LLM output, all of these things are presented in the same fluid, confident-sounding style: you have to know the material already to realize when your foot has gone through what was earlier solid flooring. That, to me, is one of their most pernicious features. I know that these things were not designed per se to glide over or hide their weak points and their mistakes, but they do a terrific job of it, and that's not really what you want. So as much as I found some parts of the Deep Research output impressive, I found its deeper research problems hard to deal with.
Generative AI is complicated. How we talk about it should be, too. When AI makes errors they’re often subtle and buried in language that is confident. The gist we get reading such outputs: Trust me. I know what I’m doing. I’m artificial intelligence. But a large language model is no more intelligent than a toaster. Why do we want to believe otherwise?
The Mechanical Turk's operators crafted an illusion of mechanical intelligence that lasted decades through often elaborate displays of gears and clockwork—visual accessories to sell the experience to eager crowds. Generative AI companies construct their own digital theater of sorts, selling the illusion of consciousness through carefully designed interfaces and human-like responses. The Turk's creators knew that visible machinery would make the illusion more convincing; GenAI developers likewise expose reasoning traces and chain of thought techniques to makes their systems appear more human-like and trustworthy. Both embrace theatrical elements to not just entertain, but convince us that we're witnessing something profound.
The False Comfort of AI Comparisons
When ChatGPT first launched, quite a few people compared generative AI to the arrival of the calculator—a technology that changed how we taught maths in schools. We’re human. We learn in part through comparison. Saying something is like another makes the strange familiar. After all, generative tools are just technology, one made not simply by us but also from us: our words, our images, and our utterances form the training data that the models use.
Some two years later, I can now task an AI agent to use a digital calculator within my web browser. How can this not be intelligence! But it isn’t. The calculator analogy failed not just because it was imperfect, but because it fundamentally misunderstood what makes AI different from previous tools. A calculator performs precise, well-defined mathematical operations—there's no ambiguity about what happens when you press '3 + 3.' But generative AI operates in the realm of probability and pattern matching, making it more like a sophisticated guessing machine than a straightforward tool.
When we try to compare generative tools to existing technologies, we inevitably focus on surface-level similarities and miss the profound differences in how these systems function. The calculator doesn't pretend to think about math; it simply computes. Generative tools, in contrast, are designed to create the illusion of thought, making them resistant to simple technological analogies.
We should look deeply at what generative AI represents outside of the comfortable familiarity of analogies and treat it as something astonishingly statistical. Otherwise, I fear the analogy we’re ultimately headed for is comparing AI to us, and that badly misrepresents what this technology actually is, what it does, and what people might take for it to mean.
How Language Shapes Our AI Perceptions
To put it bluntly, the language we use to talk about generative technologies is just plain bad. We’ve accepted the terminology that humanizes and familiarizes algorithmic processes with little protest or critical analysis. When a model commits an error, we don’t call it a failure of prediction. Instead, we say it hallucinated, something human beings do when they are confused or mistaken, not something we’d rationally associate with a machine. All because we aren’t supposed to feel as though we’re working with just a machine.
We’ve somehow collectively decided that a large language model’s ability to map and predict the most fundamental human creation of ours, that of written language, makes it something more than just numbers and predictive processing power.
Now we are awash in so-called reasoning models that can think through problems and use chain-of-thought prompting to show us their process. OpenAI’s o1 and o3 models and now DeepSeek’s free R1 model all include anthropomorphization as part of their user design. Look around on social media to see how real flesh and blood folks are talking about Deepseek. They like its response because it feels more human-like.
If you’ve used a chatbot interface like ChatGPT, you too may have found your interactions with the interface beginning to blur: please do this for me; I’d like you to take your time; thanks, that was pretty good, but you can do better. The humanization of machines isn’t a by-product here—it’s part of the package.
How We Talk About AI Matters
When a language model creates an error we call it a hallucination—a human concept for organic minds—not remotely the same as it is for synthetic systems. So-called reasoning models that use advanced techniques to chain decisions together are very good at walking through processes step-by-step; however, the “reasoning” within them isn’t anything like what we find in human beings.
I’m sure some will argue using humanist terms like hallucination and reasoning serve as useful metaphors, helping us non-technical users understand and work with these new systems. Isn’t hallucination easier to explain than the statistical mechanics of next token prediction? Yet this simplification comes at a steep cost. When we describe AI systems in humanizing terms, we create false expectations about their capabilities and their limitations. A user who believes an AI can reason might well trust its output about important decisions where human judgment is essential. The convenience of using anthropomorphic language obscures what these systems actually are and what they can reliably do.
Exploring DeepSeek’s R1 Reasoning Traces
Let’s dive into the data that’s been collected about the reasoning from DeepSeek. The folks at Nomic somehow gathered a staggering database of over 250,000 DeepSeek reasoning traces. You can use Nomic’s Atlas to analyze and see patterns of what people have prompted, but most importantly, you can quickly scan the traces to visualize the model’s response.
The more examples you view, the more you find a shallowness to them. Reasoning trace might be an apt name for these because they’re not thinking—rather summaries of a deterministic decision-making process. You can see this as DeepSeek’s R1 walks a user through a reasoning trace using a search. Here I asked the model to help structure AI policies at US R1 schools. The trace does an apt job of summarizing a dozen websites, but let’s look at its response carefully.
Looking at the webpages, webpage 1 seems to be about Greenland annexation, which is unrelated. Whoa there, the first source scanned was actually from Syracuse University’s news site leading to AI Policy Expert Discusses Changing Regulatory Landscape. That’s an error, a “hallucination.” The problem is, does the error compound the following result? How can I know? A human being would pause here, reset, and think, but a reasoning model cannot think. It has started its process and was locked in. Let’s keep going.
Webpage 2 from Stanford discusses a framework focusing on ethical concerns like privacy, bias, and environmental impact. That's useful. Well, maybe. But how is it useful? A human would put this into context, not move along to the next source, but that’s exactly what happens.
Webpage 3 is a playbook from the Manhattan Institute with principles on national security and human dignity. Some parts might apply, especially regarding security and ethical use. Maybe, but that’s pretty weak. Why are we looking at the Manhattan Institute vs. hundreds of other sources? What process brought you to this source?
The final output isn’t bad. It represents a solid overview of many of the policy discussions I’ve reviewed from R1 universities, but the point is DeepSeek wasn’t anything like human reasoning that created the output. DeepSeek didn’t “think for 7 seconds,” and didn’t ponder the sources and how they relate to the broader context of people and events and all those messy human values we carry around. It used a deterministic process that locked itself into a scan of a certain number of sources, the number of which was random. The process it shares with us is limited to what it was programmed to share based on a human development team's idea of how much they believed an audience needed to know before trusting a response.
The DeepSeek example shows how reasoning traces create a convincing illusion of machine intelligence. While the system produces seemingly logical steps and admits to some errors, it easily misses others. DeepSeek follows a predetermined pattern—scan sources, extract information, and generate a summary. That’s it. Unlike human reasoning, which can recognize when an approach isn't working and change course, these systems move forward with an entirely synthetic process without understanding any of the meaning behind its decisions.
Let’s Move Past Illusions
The illusion of AI reasoning is our Mechanical Turk moment with these powerful machine systems. But reasoning traces that mimic intelligence aren’t just a harmless interface choice—they are a deliberate design strategy that shapes how we talk about and interact with these systems.
The Mechanical Turk's ability to play chess was a hoax, but we didn't confuse computer logic with human intelligence when it began to play chess, so let’s slow down and see the forest for the trees.
We’d be wise to develop a careful vocabulary for discussing generative AI—one that acknowledges both its capabilities and limitations without relying on human analogies. Instead of reasoning traces, frame it as prediction pathways. Rather than hallucinations, call them errors. This isn't just semantic nitpicking; it's about building a framework that lets us see these tools for what they truly are.
The Mechanical Turk's illusion eventually ended, but not before shaping decades of public discourse about mechanical intelligence. I fear we may be at a similar crossroads in our evolving generative era.
Open Disclosure of AI: I used DeepSeek to generate the linked example. I also used Anthropic’s Claude to help explore connections between the Mechanical Turk and LLMs.
Thanks for a thought provoking article. The way we anthropomorphize AI (and tech in general) has some serious implications. I still struggle with my own mental models about AI and I suspect I'm not alone.
Great piece, Marc. It affirms my sense that we need analogies and metaphors different from those of the human mind. The initial enthusiastic response to the new models always imagines the gap between what the machine can do (quite impressive in this case!) and what a human would do as slight. It feels close if you imagine the machine is thinking. How hard can it be to have the model's outputs agree with reality or truth?
The answer is that it is quite hard. The model does not think in a way that fits the analogy of the human mind's processes. It does not have an understanding of reality or truth outside the vectors it uses to manipulate language. Layering on post-training to get it to answer questions more reliably is not going to fix its fundamental nature, which is grounded in probabilistic mathematics applied to language, not a human understanding of reality.