In mid December, Google Labs revealed some really cool experiments with generative AI and other types of machine learning. What really grabbed my attention is how geared toward education many of these new tools are. What’s equally fascinating is how Google is using games to foster creative exploration with this new technology and how easy it is for K12 or higher education folks to adapt and pair such activities with critical reflection to help students not only understand how parts of the underlying tech functions, but also help them build their knowledge about music, language, and the arts.
TextFX
Google used collaboration to engage users and let them see potential use cases. Their partnership with Lupe Fiasco to build TextFX is really something unique. Fiasco used the system to help him compose lyrics, but when you break down the overall system what TextFX really represents is an app to explore language and how words and concepts relate or depart from one another. It’s a really creative experiment that any educator can build assignments around to help students explore creative expression.
TextFX Use Cases
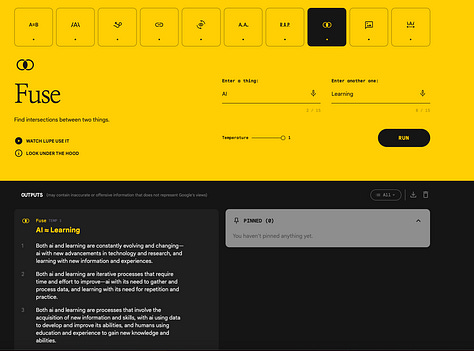
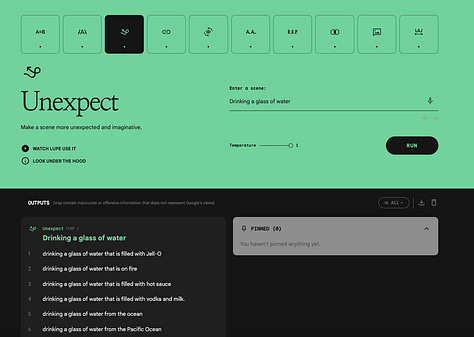
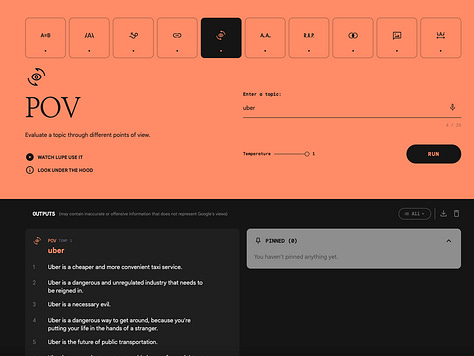
PopSign
One of the many complaints I’ve repeatedly heard about current genAI apps is that their experimental label often means developers exclude those with disabilities. This is why PopSign, a system that uses AI to help users learn American Sign Language, is impresses me. Here, we actually can see the hyped promise of the technology starting to take shape as a thoughtful tool to help those who’d like to learn sign language.
NotebookLM
I’ve written about Google’s NotebookLM before and am still on the fence if Google can actually get Retrieval Augmented Generation to work. Having an LLM talk to your data without it hallucinating or making up facts is the veritable space race Google, Microsoft, Amazon, and many others have entered into. The app is now freely available and they’ve upgraded it to their ChatGPT-level version of Gemini. What makes NotebookLM stand out is its size—you can upload 4,000,000 words per notebook! If developers ever figure out RAG, then there are a seemingly endless number of use cases for talking to your data.
Google’s Arts and Culture Experiments
All of the apps above are great, but for educators, Google’s Arts and Culture experiments are where the relationship between AI + ML really shines. It’s clear Google spent a great deal of time building out these apps and working with art history experts and educators to explore use cases. The implication here is astounding when you consider Google built all of these apps just to help learners use AI+ML to explore a single discipline and how easily these existing apps can be used to teach students basic AI literacy.
Say What You See—An Introduction to Prompting
One of the most challenging aspects of teaching someone how to prompt an LLM is understanding that prompting is a highly iterative process. A user needs practice with a number of knowledges to get a competent output. This is where Say What You See shines. It teaches users the basis for prompting an LLM for image generation in progressively challenging rounds that require more focus and accuracy. What educators have noticed and tried to convey to learners is they’re not going to achieve an amazing response without first knowing what they want an output to be. To do that, they need:
Rhetorical knowledge: knowing how to frame a question, query, or prompt.
Content knowledge: understanding the subject they are prompting an LLM
Context knowledge: being able to use natural language to describe with great detail the medium, subject, and put them into context to get a solid response
Say What You See is challenging even for me. I cannot get past the 6th level. In part because I don’t have enough content knowledge about art history to effectively write a prompt to generate a coherent output that meets the needs of the system. In other words, the thing many of educators have been telling our students for the past year becomes strikingly clear—you cannot prompt a system to give you an answer if you don’t know enough about the subject or details!
ArtRemix—More Prompting Opportunities
One grub I have with these experiments is they’re just sort of dumped into a disorganized pool of resources with some vague notion of how they might be used by the public. Years in education as a teacher has given me a keen eye toward scaffolding resources and seeing how they might fit in helping a learner understand, then creatively explore a skill. To me, ArtRemix is the logical companion to Say What You See. After a user learns the complexity of prompting for coherence, they can then be exposed to prompting for creative expression.
Odd One Out—Find the AI Generated Image
The implication for image generation technology to warp digital space with propaganda, deep fakes, misinformation is one of the alarming real-world harms occurring now. It’s a heavy topic, and not one that’s easy to broach with k12 or some students in higher education. But Odd One Out lets users explore digital trickery by asking them to make an educated guess about what image out of a series of four was generated. Like I said with Say What You See, the user quickly discovers they need some level of familiarity with their subject in order to have a chance at the game.
Guess The Line—Understanding How Neural Networks Function
One of my favorite games that I play with faculty and students to get them to consider how machine learning and neural networks function is Google’s Quick, Draw! The timed doodling game where a user tries to draw an image and the AI tries to guess it based off of its training data is an excellent and fast opportunity to show how the technology functions—input, prediction, then output. The broader point I attempt to convey is that an LLM is just a very sophisticated guessing game!
Guess The Line appears to be an even more sophisticated version of Quick, Draw! It’s certainly much more challenging and really requires the user to engage all the knowledges discussed her to get a coherent output by drawing three images in less than 60 seconds. I think it is a really sharp teaching tool for students to explore neural networks.
Cultural Icons—Learning with AI
Having an LLM and image generator teach users to hone their ability to recognize cultural icons is a thoughtful gamified way to explore cultural literacy. You can play a variety of modes within the game, from finding the cultural icon from a series of images and getting clear explanations about why your selection met the criteria or didn’t quite rise to icon status, to randomly quizzing yourself about a topic.
The interface is simple and it blends a chat feature that you can have a limited back and forth with quite seamlessly.
Blob Opera—Pure Fun Exploring Music Generation
My kids love Blob Opera! This experiment uses machine learning to have a series of blobs sing a generated song. All a user needs to do is drag their cursor over a blob and create a song. It’s a great way to creatively explore how music generation functions.
The Big Picture—Generative AI Can Help Students Learn
Since 2010, Google has published over 1600 experiments using a variety of AI, LM, and other digital tools. It’s truly a testament to see how they’ve explored so many use cases and applications over the years. Most of these tools can be found through Google Labs, but the main experiments page is also worth a deep dive.
What really grabs my attention when exploring Google's array of AI experiments is the sheer range of creative educational possibilities. From songwriting and language exploration with TextFX to learning ASL with PopSign to seeing how to prompt with image generators, students can learn by doing - actively engaging with various models to foster self-expression and build critical AI literacy.
2024 will be a messy year for generative AI, so having a range of go-to activities that educators can call on to teach students some aspects of this technology is most welcome. I’d really like to see them move more toward scaffolding this material for k-12 and even higher edu audiences. To me, that’s the missing link here. The systems are already in place, we just need more creative application and engaged pedagogy to see its full potential as learning tools.
These are all interesting. The proliferation of AI applications, however, are making it really difficult for anyone to feel like they have a handle on what are the most useful. Further, due to issues with how our school allows permissions for various sites and tools, we do not have access to Google Labs on our school account - to take advantage of even playing around with them requires going through your personal account. While none of this is especially difficult, it does raise the bar for rolling them out to students and teachers during professional development. The lack of coordination and speed with which new AI platforms are being released is a little overwhelming. I am curious to see how things play out over the course of the year and whether any major player becomes the dominant tool in the edtech market. Simplicity and ease of use will have to be at the top of the list if schools are ever to truly embrace using AI in classrooms. At the moment, it feels like everything is piecemeal and it's hard to get a sense of what is worth spending the time learning how to use.